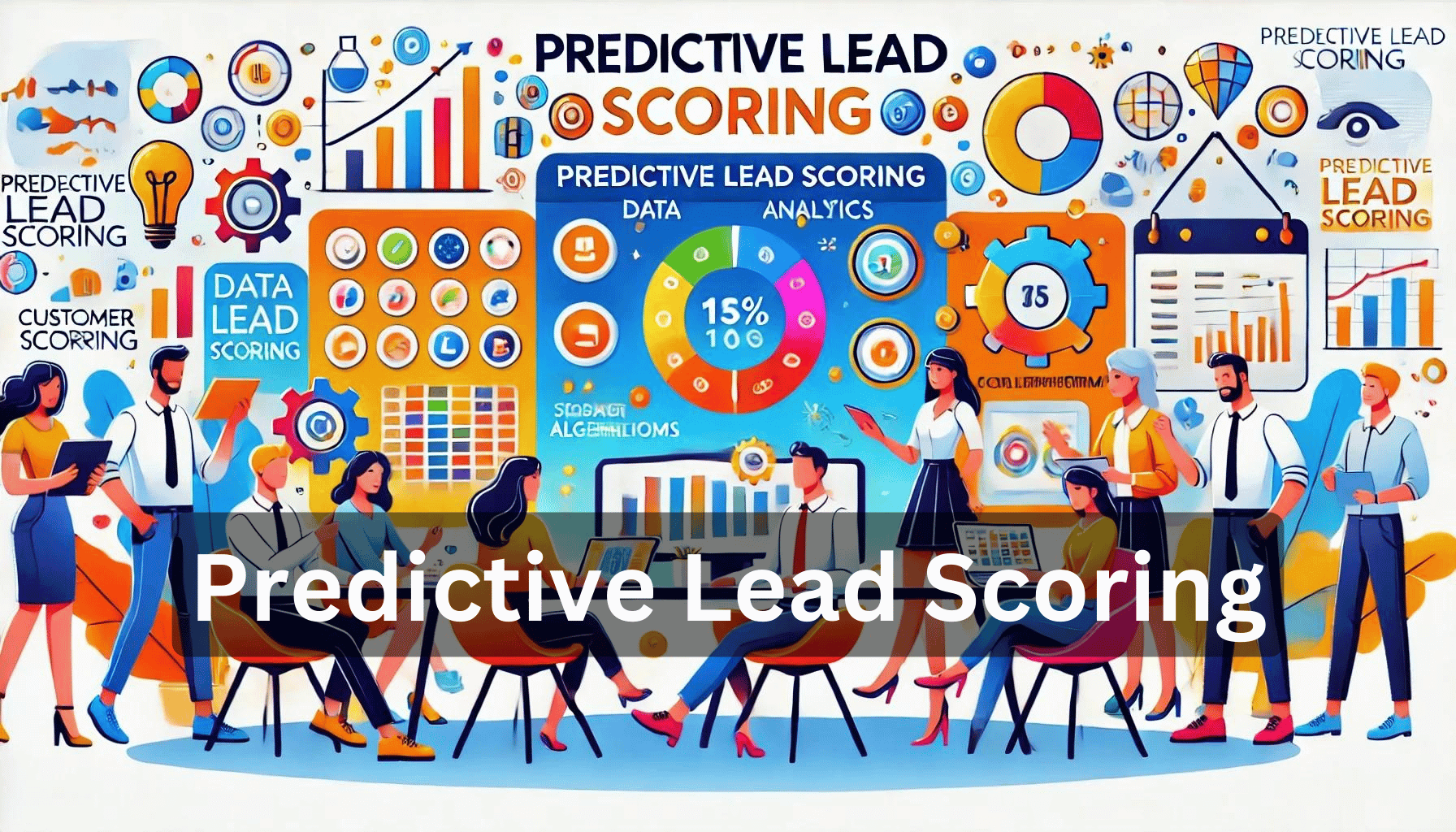
Unlocking the Potential of Predictive Lead Scoring with HubSpot
Unlocking Sales Potential with Predictive Lead Scoring: Benefits, Uses, and HubSpot Integration
In today’s competitive business landscape, effectively managing and prioritizing leads is crucial for sales success. Predictive lead scoring is a powerful tool that leverages data-driven insights to identify and prioritize high-quality leads, ensuring your sales and marketing teams focus their efforts where they matter most. This article delves into the benefits and workings of predictive lead scoring, explores its integration with HubSpot, and provides a comprehensive guide on implementing this technology to boost your sales performance.
Article Outline
- What is Predictive Lead Scoring?
- How Does Predictive Lead Scoring Work?
- Benefits of Using Predictive Lead Scoring
- Integrating Predictive Lead Scoring with HubSpot
- Predictive Lead Scoring vs. Traditional Lead Scoring
- Implementing Predictive Lead Scoring in Your Business
- How to Build an Effective Predictive Lead Scoring Model
- Common Challenges and Solutions in Predictive Lead Scoring
- Real-World Examples of Predictive Lead Scoring Success
- Future Trends in Predictive Lead Scoring Technology
What is Predictive Lead Scoring?
Predictive lead scoring is a data-driven approach that uses machine learning algorithms to analyze thousands of data points, identifying leads most likely to convert into customers. Unlike traditional lead scoring, which often relies on subjective criteria, predictive lead scoring leverages historical data and predictive modeling algorithms to provide a more accurate assessment of lead quality.
Here is a video to help you better understand Predictive Lead Scoring:
How Does Predictive Lead Scoring Work?
Predictive lead scoring works by using machine learning to analyze historical data and identify patterns that indicate a lead's likelihood to convert. The algorithm considers various data points, such as demographic information, behavior on your website, interaction with marketing materials, and previous purchasing history. By processing this data, the predictive lead scoring model assigns a score to each lead, helping sales teams prioritize leads based on their likelihood to buy.
For an automated approach to lead prospecting, consider integrating tools like SalesMind's LinkedIn Prospecting Automation, which can enhance your lead generation process by identifying and targeting high-quality leads on LinkedIn.
Here is a flowchart to help you better visualize Predictive Lead Scoring:

Benefits of Using Predictive Lead Scoring
Predictive lead scoring offers numerous benefits, including:
- Increased Efficiency: By automating the lead scoring process, sales teams can focus on leads most likely to convert, reducing time spent on unqualified leads.
- Improved Conversion Rates: With accurate lead scores, marketing and sales teams can tailor their approaches, leading to higher conversion rates.
- Better Alignment Between Sales and Marketing: Predictive lead scoring provides a common framework for evaluating leads, fostering better collaboration between marketing and sales teams.
Integrating Predictive Lead Scoring with HubSpot
HubSpot's predictive lead scoring feature leverages the power of predictive analytics to enhance your lead scoring efforts. By integrating predictive lead scoring into your HubSpot CRM, you can automate lead scoring, prioritize leads more effectively, and improve overall sales performance. HubSpot’s predictive lead scoring uses machine learning to analyze your lead data and assign scores based on the likelihood to convert.
Predictive Lead Scoring vs. Traditional Lead Scoring
Traditional lead scoring often relies on manual processes and subjective criteria, leading to potential human error and inconsistencies. In contrast, predictive lead scoring uses machine learning algorithms to analyze vast amounts of data, providing a more accurate and objective assessment of lead quality. Understanding the differences between these approaches can help you make an informed decision about which method to implement in your business.
Implementing Predictive Lead Scoring in Your Business
To successfully implement predictive lead scoring, follow these steps:
- Define Your Objectives: Clearly outline your goals and what you hope to achieve with predictive lead scoring.
- Gather Data: Collect and organize historical data on your leads, including demographic information, behavioral data, and past interactions.
- Choose the Right Tools: Select a predictive lead scoring software that integrates with your existing CRM and marketing automation tools.
- Build and Test Your Model: Use your data to create a predictive lead scoring model and test its accuracy before full implementation.
- Train Your Team: Ensure your sales and marketing teams understand how to use the predictive lead scoring system effectively.
To explore more tools that can aid in this process, visit SalesMind.
How to Build an Effective Predictive Lead Scoring Model
Building a predictive lead scoring model involves several key steps:
- Data Collection: Gather comprehensive data on your leads, including demographic details, behavior on your website, and engagement with marketing materials.
- Feature Selection: Identify the most relevant data points that indicate a lead's likelihood to convert.
- Model Training: Use machine learning algorithms to train your model on historical data, optimizing it for accuracy.
- Validation and Testing: Validate your model with a separate dataset to ensure it accurately predicts lead conversion.
- Implementation: Integrate your predictive lead scoring model into your CRM and marketing automation systems.
For a seamless integration, consider using SalesMind's CRM and marketing automation tools.
Common Challenges and Solutions in Predictive Lead Scoring
While predictive lead scoring offers many benefits, it also presents some challenges:
- Data Quality: Ensuring your data is accurate and up-to-date is crucial for the effectiveness of your predictive lead scoring model. Regularly clean and update your data to maintain its quality.
- Model Accuracy: Continuously monitor and refine your predictive lead scoring model to ensure it remains accurate over time.
- Team Buy-In: Secure buy-in from your sales and marketing teams by demonstrating the value of predictive lead scoring and providing proper training.
Real-World Examples of Predictive Lead Scoring Success
Many companies have successfully implemented predictive lead scoring to improve their sales performance. For example, a SaaS company used predictive lead scoring to prioritize leads, resulting in a 30% increase in conversion rates. Another company in the e-commerce sector leveraged predictive lead scoring to identify high-quality leads, leading to a significant boost in sales and revenue.
Future Trends in Predictive Lead Scoring Technology
The future of predictive lead scoring lies in the continued advancement of machine learning and big data technologies. As these technologies evolve, predictive lead scoring models will become more accurate and sophisticated, enabling businesses to better identify and prioritize high-quality leads. Additionally, the integration of predictive lead scoring with other sales and marketing tools, such as CRM and marketing automation platforms, will further enhance its effectiveness.
Enhancing Lead Generation with Predictive Lead Scoring
One of the key areas where predictive lead scoring can make a significant impact is in lead generation. By using predictive modeling to analyze potential customers, businesses can create a profile based on past successful leads. This profile, or “ideal customer,” helps in identifying the warmest leads that are most likely to convert. As a result, the lead generation process becomes more efficient, focusing efforts on quality leads rather than the sheer number of leads.
Leveraging Big Data and Predictive Analytics
The power of predictive lead scoring is amplified when combined with big data and predictive analytics. By analyzing thousands of data points, predictive lead scoring uses machine learning algorithms to predict future buying behaviors. This data-driven approach not only increases lead quality but also helps in automating the lead scoring process, reducing the chances of human error and ensuring that no high-potential lead slips through the cracks.
Practical Steps to Implement Predictive Lead Scoring
- Integration with CRM and Marketing Automation: Tools like HubSpot CRM and Salesforce are essential for the seamless integration of predictive lead scoring. These platforms allow for the automation of lead scoring efforts and provide a centralized location for all lead-related data.
- Continuous Model Refinement: The predictive lead scoring model should be continuously refined and updated with new data points to maintain its accuracy. This ongoing process ensures that the lead scoring system adapts to changing market conditions and customer behaviors.
- Training and Support: Providing adequate training for your sales and marketing teams on how to use predictive lead scoring software is crucial. This training should include understanding predictive lead scoring, using predictive lead scoring software, and interpreting the scores for effective decision-making.
Overcoming Challenges in Predictive Lead Scoring
To maximize the benefits of predictive lead scoring, it is important to address common challenges. Ensuring high-quality data, regularly updating the predictive lead scoring model, and fostering collaboration between sales and marketing teams are critical steps. Additionally, using advanced predictive modeling algorithms to analyze data can enhance the accuracy and reliability of the scoring process.
The Role of Machine Learning in Predictive Lead Scoring
Machine learning is at the core of predictive lead scoring. By leveraging machine learning models, businesses can analyze vast amounts of historical data to predict future lead behaviors. This process involves using predictive modeling algorithms to identify patterns and trends that indicate a lead's likelihood to convert. The result is a more efficient and effective lead scoring system that prioritizes leads based on their potential to become customers.
Case Study: HubSpot’s Predictive Lead Scoring
HubSpot’s predictive lead scoring feature is a prime example of how predictive lead scoring works in practice. By integrating predictive analytics into its CRM, HubSpot enables businesses to automate lead scoring and prioritize leads more effectively. This integration not only improves conversion rates but also enhances the overall efficiency of sales and marketing teams. HubSpot's predictive lead scoring may be particularly beneficial for businesses looking to streamline their lead scoring process and focus on quality leads.
Conclusion
Predictive lead scoring is a powerful tool that leverages data-driven insights and machine learning to prioritize leads based on their likelihood to convert. By integrating predictive lead scoring with tools like HubSpot and Salesforce, businesses can automate the lead scoring process, improve lead quality, and enhance overall sales performance. Understanding and implementing predictive lead scoring can unlock significant sales potential, providing a competitive edge in today’s market.
Key Takeaways
- Predictive lead scoring is a data-driven approach that uses machine learning algorithms to analyze data and identify high-quality leads.
- By integrating predictive lead scoring with tools like HubSpot, businesses can automate lead scoring and prioritize leads more effectively.
- Predictive lead scoring offers numerous benefits, including increased efficiency, improved conversion rates, and better alignment between sales and marketing teams.
- Implementing predictive lead scoring involves gathering data, building a model, and training your team to use the system effectively.
- Future trends in predictive lead scoring technology will focus on the continued advancement of machine learning and big data, leading to more accurate and sophisticated models.
- Leveraging big data and predictive analytics enhances the power of predictive lead scoring, making the lead generation process more efficient.
- Continuous refinement of the predictive lead scoring model and adequate training for sales and marketing teams are crucial for maximizing its benefits.
For more information on how to integrate predictive lead scoring into your sales strategy, explore the solutions offered by SalesMind. Sign up for their tools here and transform your lead generation process today.
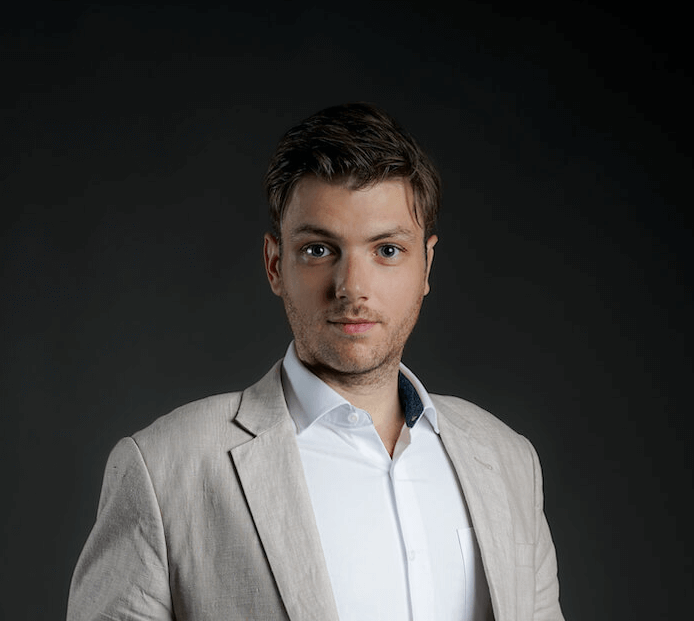
Julien Gadea specializes in AI prospecting solutions for business growth. Empowering businesses to connect with their audience with SalesMind AI tools.
Julien Gadea
Subscribe for the latest on Artificial Intelligence in sales
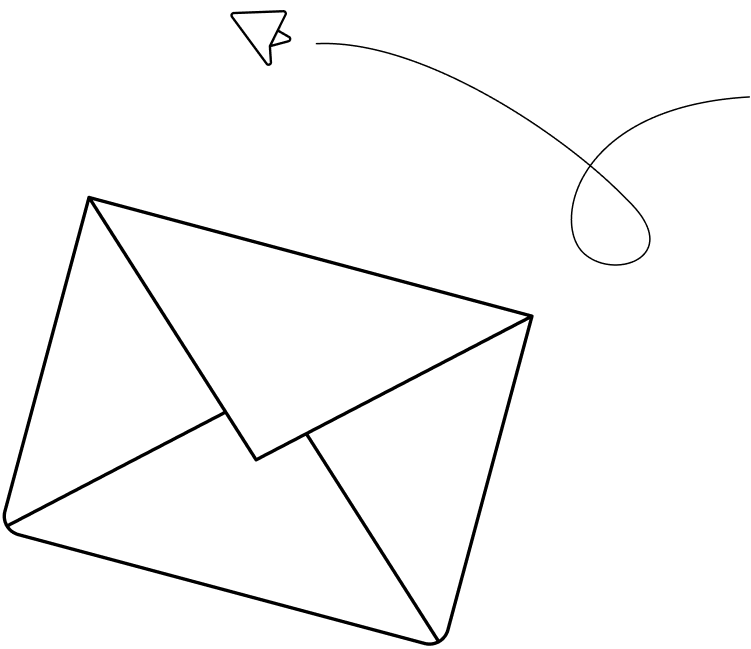