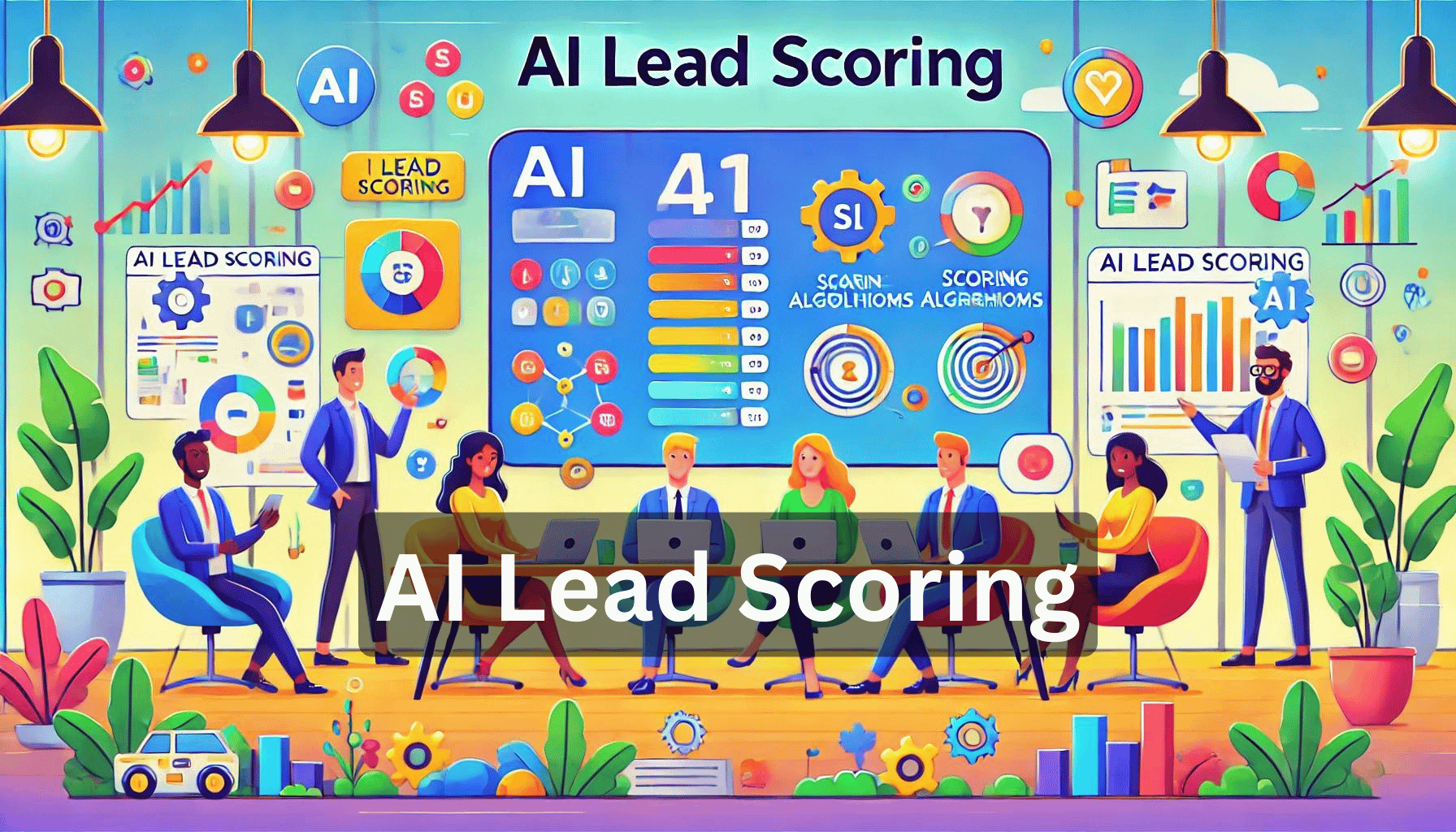
Mastering AI Lead Scoring: Your Ultimate Guide to Predictive Lead Scoring with AI
Unlocking Sales Potential with Predictive Lead Scoring Using AI
Summary: In the evolving landscape of sales and marketing, leveraging AI for predictive lead scoring can be a game-changer. This article delves into the intricacies of predictive lead scoring with AI, explaining how it can revolutionize lead management and significantly enhance your sales outcomes. It's a must-read for marketing and sales professionals aiming to optimize their strategies and drive better results.
Outline
- What is Predictive Lead Scoring?
- How Does AI Enhance Lead Scoring?
- Benefits of AI-Powered Predictive Lead Scoring
- Traditional vs. Predictive Lead Scoring: What’s the Difference?
- How Does Machine Learning Improve Lead Scoring?
- Implementing Predictive Lead Scoring in Your Organization
- Key Features of Effective AI Lead Scoring Models
- Challenges and Solutions in AI Lead Scoring
- Case Studies: Success Stories of AI Lead Scoring
- Future Trends in Predictive Lead Scoring
What is Predictive Lead Scoring?
Predictive lead scoring is a sophisticated method used to determine the potential value of a lead by analyzing various data points. Unlike traditional lead scoring, which relies on static criteria and manual inputs, predictive lead scoring leverages AI and machine learning algorithms to assess leads based on their likelihood to convert.
Using predictive lead scoring, businesses can analyze past interactions, demographic information, and behavioral data to predict future actions. This approach offers a dynamic and accurate scoring system that adapts to changes in data and market trends.
How Does AI Enhance Lead Scoring?
AI enhances lead scoring by automating the process and providing a more accurate assessment of lead quality. AI lead scoring uses advanced machine learning algorithms to analyze vast amounts of data, identifying patterns and correlations that would be impossible for humans to detect manually.
By using AI, businesses can ensure that their lead scoring system is constantly learning and improving. This leads to better predictions and more effective lead management strategies. AI can help marketing and sales teams focus on the most promising leads, thereby improving efficiency and conversion rates.
Benefits of AI-Powered Predictive Lead Scoring
Implementing AI-powered predictive lead scoring offers numerous benefits:
- Accuracy: AI-driven models provide more precise lead scores by considering a wider range of data points and learning from new data.
- Efficiency: Automating the lead scoring process saves time and resources, allowing sales and marketing teams to focus on high-priority tasks.
- Scalability: AI lead scoring systems can handle large volumes of data, making them suitable for businesses of all sizes.
- Real-time insights: AI provides real-time lead scoring, enabling quicker decision-making and more agile marketing strategies.
Here is a video comparing AI Lead Scoring to Rule-Based Scoring:
Traditional vs. Predictive Lead Scoring: What’s the Difference?
Traditional lead scoring relies on predefined criteria and manual scoring methods. This approach can be subjective and often lacks the flexibility to adapt to new data. On the other hand, predictive lead scoring uses AI and machine learning to continuously refine and improve the scoring model.
Traditional lead scoring can be effective in simpler scenarios, but it falls short in complex environments where data volume and variety are high. Predictive lead scoring, with its dynamic nature, can handle these complexities, providing more reliable and actionable insights.
How Does Machine Learning Improve Lead Scoring?
Machine learning improves lead scoring by analyzing large datasets to identify patterns and trends. It uses algorithms to learn from historical data, making predictions about future lead behavior. This allows businesses to:
- Predict Conversion Probability: Machine learning algorithms can predict the likelihood of a lead converting based on past behavior and characteristics.
- Identify High-Value Leads: By analyzing data patterns, machine learning can highlight leads with the highest potential value, ensuring sales efforts are directed where they are most needed.
- Adapt to Changes: Machine learning models continuously learn from new data, allowing the lead scoring system to evolve and improve over time.
Implementing Predictive Lead Scoring in Your Organization
Implementing predictive lead scoring involves several steps:
- Data Collection: Gather data from various sources, including CRM systems, marketing automation platforms, and customer interactions.
- Model Development: Use machine learning algorithms to develop a predictive model based on historical data.
- Integration: Integrate the predictive model into your existing lead management systems.
- Testing and Validation: Test the model to ensure accuracy and refine it based on feedback and new data.
- Continuous Improvement: Continuously monitor and update the model to ensure it remains effective.
Key Features of Effective AI Lead Scoring Models
Effective AI lead scoring models share several key features:
- Data Integration: They integrate data from multiple sources to provide a comprehensive view of each lead.
- Adaptability: They continuously learn and adapt to new data and changing market conditions.
- Transparency: They provide clear explanations for lead scores, helping teams understand the reasoning behind the scores.
- Scalability: They can handle large volumes of data and scale with the growth of the business.
Challenges and Solutions in AI Lead Scoring
While AI lead scoring offers many benefits, it also comes with challenges:
- Data Quality: Poor data quality can lead to inaccurate predictions. Ensuring clean, accurate data is crucial.
- Complexity: Implementing AI systems can be complex and require specialized knowledge. Partnering with experienced vendors can help.
- Resistance to Change: Teams may be resistant to adopting new technologies. Providing training and demonstrating the benefits can mitigate this.
Common Challenges and Solutions in AI Lead Scoring
Case Studies: Success Stories of AI Lead Scoring
Many businesses have successfully implemented AI lead scoring, achieving significant improvements in lead management and sales outcomes. For instance, SalesMind AI’s LinkedIn prospecting automation system has helped companies prioritize leads more effectively, resulting in higher conversion rates and increased revenue.
SalesMind AI’s solutions have consistently provided actionable insights, helping sales teams focus on the best leads and close deals faster. Their tools analyze customer data comprehensively, enabling better decision-making and enhanced lead management.
Future Trends in Predictive Lead Scoring
The future of predictive lead scoring lies in further advancements in AI and machine learning technologies. As these technologies continue to evolve, we can expect:
- Increased Accuracy: Improved algorithms will provide even more accurate lead scores.
- Better Integration: Enhanced integration with other marketing and sales tools will streamline processes and improve efficiency.
- Personalization: AI will enable more personalized lead scoring, taking into account individual lead preferences and behaviors.
The Role of Lead Scoring Software in AI-Powered Predictive Lead Scoring
Lead scoring software plays a crucial role in enabling predictive lead scoring with AI. These tools integrate various data sources and apply AI models to provide real-time insights into lead quality. By automating the lead scoring process, these software solutions help businesses manage leads more efficiently and effectively.
AI lead scoring software can analyze vast amounts of data from CRM systems, social media, and other digital touchpoints. This comprehensive analysis allows businesses to identify the best leads and tailor their marketing and sales efforts accordingly. The AI-powered lead scoring models used in these tools continuously learn and adapt, ensuring that lead scoring remains accurate and relevant over time.
Enhancing Lead Quality with AI-Powered Lead Scoring
Improving lead quality is one of the primary benefits of using AI-powered lead scoring. By analyzing a wide range of data points, AI can provide a more nuanced understanding of lead potential. This leads to better-targeted marketing campaigns and more efficient sales strategies.
AI-powered lead scoring helps businesses focus on leads that are most likely to convert, thereby increasing the efficiency of sales and marketing teams. It also provides insights into which leads may require more nurturing and which are ready for immediate follow-up. This level of detail in lead management ensures that every lead is handled appropriately, maximizing the chances of conversion.
Why AI-Powered Lead Scoring is Essential for Modern Lead Generation
In today's competitive market, effective lead generation requires more than just collecting contact information. It involves understanding the potential of each lead and tailoring marketing and sales efforts to maximize conversion rates. AI-powered lead scoring provides the insights needed to make informed decisions about lead management.
By using AI to analyze and score leads, businesses can ensure that they are focusing their resources on the most promising prospects. This not only improves lead quality but also enhances the overall efficiency of the lead generation process. AI lead scoring offers a significant advantage over manual scoring methods, providing real-time, data-driven insights that drive better results.
The Benefits of Using Predictive Lead Scoring with AI
Using predictive lead scoring with AI offers numerous advantages for businesses:
- Improved Accuracy: AI models provide more accurate lead scores by analyzing a wide range of data points.
- Increased Efficiency: Automating the lead scoring process saves time and resources, allowing teams to focus on high-priority tasks.
- Better Lead Management: AI provides detailed insights into lead quality, helping businesses manage leads more effectively.
- Higher Conversion Rates: By focusing on the best leads, businesses can improve their conversion rates and drive more revenue.
In conclusion, AI-powered predictive lead scoring is a powerful tool for modern businesses looking to enhance their lead generation and management strategies. By leveraging the capabilities of AI, companies can gain deeper insights into lead quality, improve their marketing and sales efforts, and ultimately achieve better results. Embracing this technology is essential for staying competitive in today's data-driven market.
For those looking to integrate AI into their lead scoring processes, consider exploring solutions like SalesMind AI’s LinkedIn prospecting automation or sign up for a free trial of our AI-powered tools here.
Key Takeaways
- Predictive lead scoring with AI is a powerful tool for improving lead management and sales outcomes.
- AI enhances lead scoring by providing more accurate, efficient, and scalable solutions.
- Implementing predictive lead scoring involves data collection, model development, integration, testing, and continuous improvement.
- Effective AI lead scoring models integrate data, adapt to changes, provide transparency, and scale with the business.
- Overcoming challenges in AI lead scoring requires ensuring data quality, managing complexity, and addressing resistance to change.
- Success stories and future trends indicate that AI lead scoring will continue to evolve and provide even greater benefits.
In conclusion, leveraging AI for predictive lead scoring can significantly enhance your lead management strategy, providing accurate and actionable insights that drive better sales outcomes. Embracing this technology can give your business a competitive edge in today's data-driven market.
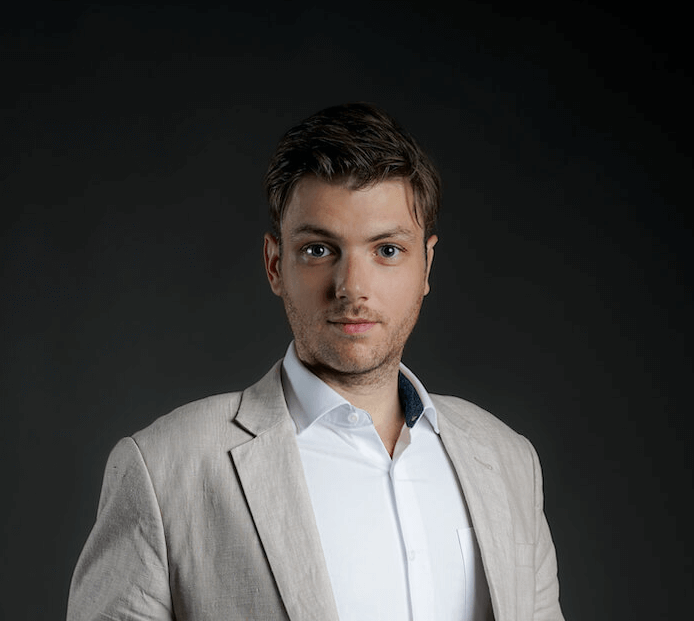
Julien Gadea specializes in AI prospecting solutions for business growth. Empowering businesses to connect with their audience with SalesMind AI tools.
Julien Gadea
Subscribe for the latest on Artificial Intelligence in sales
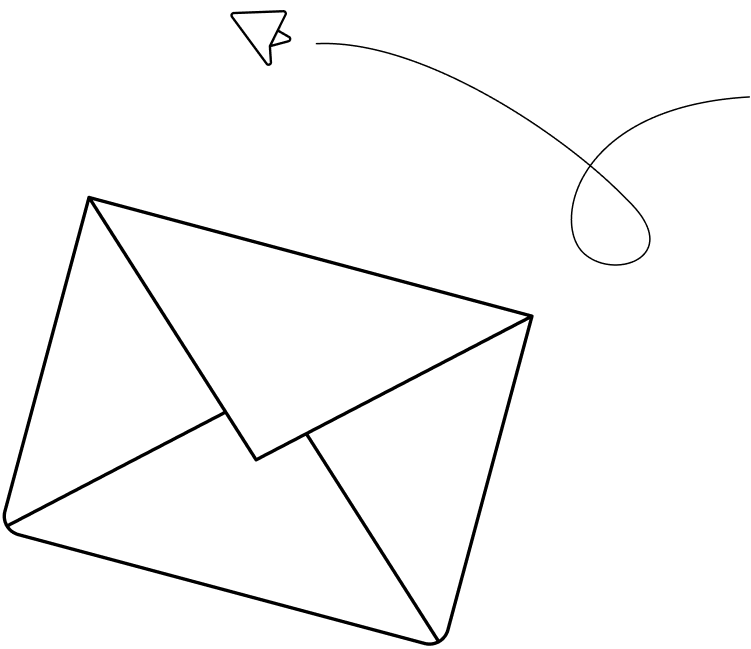